The impact of chronic diseases on public health requires new approaches to healthcare. Among these, strategies based on autonomous and miniaturized sensors capable of acquiring and interpreting biological signals reflecting the health status of patients (e.g., cardiac or respiratory activity) are particularly promising. The design of these devices must combine high computing power with extreme energy efficiency. In our project we address this challenge by proposing methodologies for co-optimizing hardware platforms and software.
Biosensor applications consist of two phases: the first dedicated to the calculation of medically relevant indicators from the acquisitions, and the second to their interpretation. Each of these phases is characterized by dedicated algorithms, offering different opportunities for optimization and hardware acceleration. We are focussing on new methodologies in the field of incremental classification for the extraction of indicators, on the customization of artificial intelligence algorithms to interpret them, and on the design of heterogeneous digital architectures to support these two tasks, taking into account their particularities.
We are developing three medical applications, where the automated interpretation of bio-signals can have a particular impact on the quality of diagnosis and prognosis: the monitoring of patients suffering from epileptic episodes, chronic cough, and knee cartilage lesions.
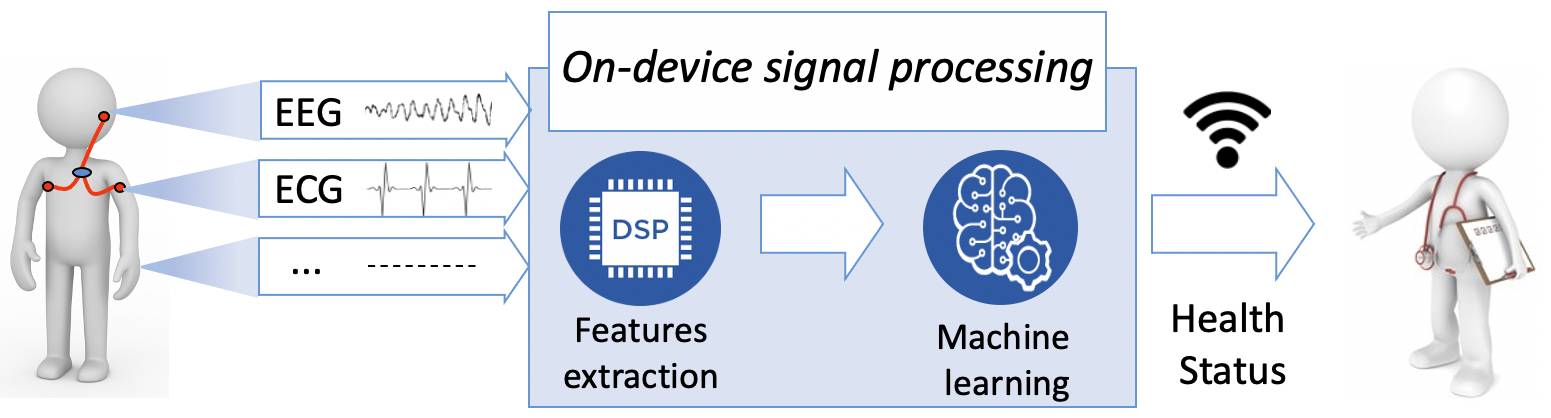
Multi-parametric Health Companions (MHCs) are unobtrusive and ultra-low-power devices, able to acquire and interpret bio-signals for health monitoring. Key to MHCs capabilities, and the focus of the Edge-Companions project, is the distributed and personalized processing of acquired inputs, in which features of clinical relevance are extracted and interpreted.

Related Publications
Keep All in Memory with Maxwell: a Near-SRAM Computing Architecture for Edge AI Applications | ||||
Eggermann, Grégoire Axel; Ansaloni, Giovanni; Atienza Alonso, David | ||||
2025-04-25 | International Symposium on Quality Electronic Design | ![]() | ![]() | |
ACE: Automated optimization towards iterative Classification in Edge health monitors | ||||
Yuxuan Wang; Lara Orlandic; Simone Machetti; Giovanni Ansaloni; David Atienza | ||||
2024-09-25 | IntIEEE Transactions on Biomedical Circuits and Systems | Early Access | ![]() |